NEWS
Rakuten Institute of Technology at the 2018 IEEE International Conference on Big Data
On December 10th to 13th, Yiu-Chang Lin, a researcher from Rakuten Institute of Technology’s Boston office, attended the 2018 IEEE International Conference on Big Data in Seattle, USA. There he presented a paper named E-commerce Product Query Classification Using Implicit User’s Feedback from Clicks at the Industry & Government session.
The 2018 IEEE International Conference on Big Data provides a leading forum for disseminating the latest results in Big Data Research, Development, and Applications. The conference collects high-quality original research papers (and significant work-in-progress papers) in any aspect of Big Data with emphasis on 5Vs (Volume, Velocity, Variety, Value and Veracity), including the Big Data challenges in scientific and engineering, social, sensor/IoT/IoE, and multimedia (audio, video, image, etc.) big data systems and applications.
Query understanding (QU) is core to search engines to infer the precise intent expressed in users’ queries and retrieve relevant content to improve user’s satisfaction and e-commerce conversion rates. A first level of query understanding includes query classification (QC) defined as the task of classifying queries into single or multiple predefined target categories. QC can boost relevance in content search by predicting which category the query belongs to and passing the hypothesis to the search engine as ranking signals. Such capability is crucial, especially in the e-commerce domain where content search is mostly directed to specific products that are typically categorized into a taxonomy tree.
Rakuten Institute of Technology at the 2018 IEEE International Conference on Big Data
2018/12/19EVENTS
On December 10th to 13th, Yiu-Chang Lin, a researcher from Rakuten Institute of Technology’s Boston office, attended the 2018 IEEE International Conference on Big Data in Seattle, USA. There he presented a paper named E-commerce Product Query Classification Using Implicit User’s Feedback from Clicks at the Industry & Government session.
The 2018 IEEE International Conference on Big Data provides a leading forum for disseminating the latest results in Big Data Research, Development, and Applications. The conference collects high-quality original research papers (and significant work-in-progress papers) in any aspect of Big Data with emphasis on 5Vs (Volume, Velocity, Variety, Value and Veracity), including the Big Data challenges in scientific and engineering, social, sensor/IoT/IoE, and multimedia (audio, video, image, etc.) big data systems and applications.
Query understanding (QU) is core to search engines to infer the precise intent expressed in users’ queries and retrieve relevant content to improve user’s satisfaction and e-commerce conversion rates. A first level of query understanding includes query classification (QC) defined as the task of classifying queries into single or multiple predefined target categories. QC can boost relevance in content search by predicting which category the query belongs to and passing the hypothesis to the search engine as ranking signals. Such capability is crucial, especially in the e-commerce domain where content search is mostly directed to specific products that are typically categorized into a taxonomy tree.
For e-commerce search queries, users typically search for either a specific product or a category of products. In both cases, a query can be associated with a category label that belongs to a taxonomy tree describing the items in the catalog. However, product-related search queries are typically short, ambiguous, and continuously changing depending on seasonal trends and the introduction of new products over time. Traditional supervised approaches to e-commerce QC are not feasible due to the high cost of manual annotation and the high volume of traffic on e-commerce search engines. In the presented paper, the researchers introduce an unsupervised method to collect large amounts of query classification data using user’s implicit click feedback, compare different state-of-the-art text classifiers, and demonstrate that an ensemble of linear SVMs models achieves outstanding performance.
For more information on the conference, see the link below.
2018 IEEE International Conference on Big Data
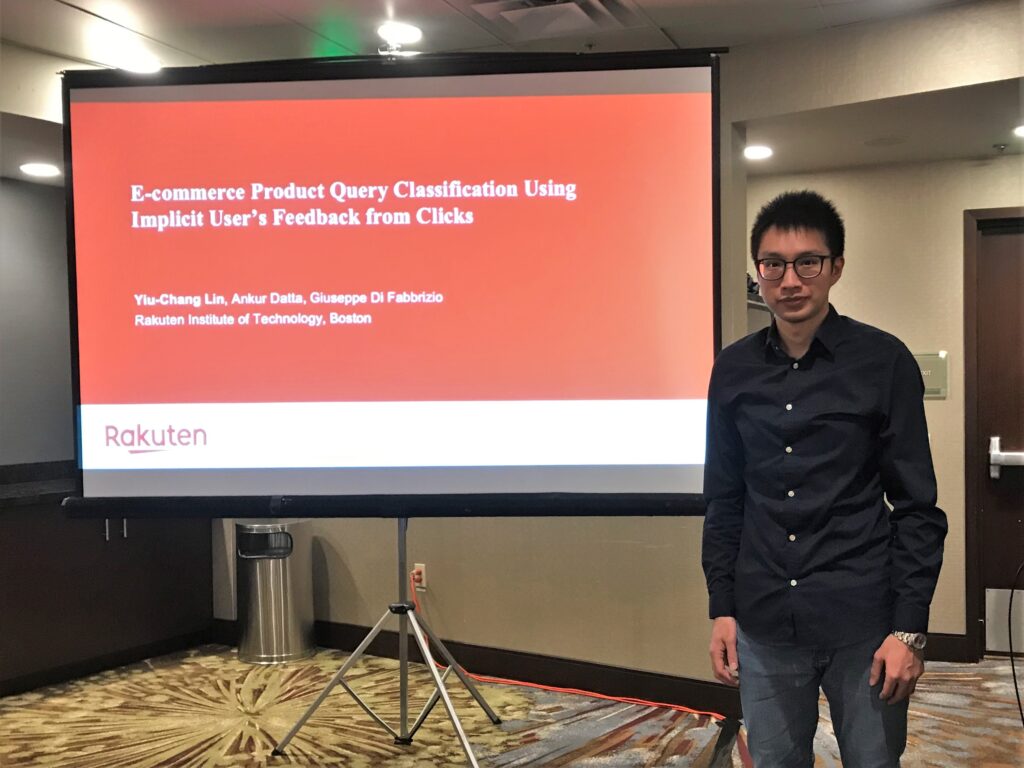