NEWS
Tianyu Li presents at IEEE International Conference on Data Mining
RIT’s Research Scientist, Tianyu Li attended the IEEE International Conference on Data Mining (ICDM) held at China’s National Convention Center in Beijing on November 9. ICDM continues to be one of the most prestigious and competitive conferences in the field. This year, out of a total of 1,046 submissions spanning 56 countries, ICDM accepted 95 regular and 99 short papers—among them Li’s paper, “Learning Classifiers on Positive and Unlabeled Data with Policy Gradient”. The paper proposes a reinforcement learning framework, in which a classifier is learned from positive (P) and unlabeled (U) examples by interacting with a policy network. The interactive learning between policy and classifier in the framework makes use of U data in a more effective manner and trains a more generalized classifier in an end-to-end fashion. Li garnered praise and inspired discussion among many of the other researchers.
Additionally, as a conference that covers a wide range of subjects, Li joined sessions in deep learning, transfer and interpretable learning, and served as one of the chairs on recommender systems, a critical field of study among RIT’s researchers. He underlined that he found the environment and caliber of the researchers enlightening, helping provide him some future directions for his ongoing projects with RIT as he continues to address the PU learning problem (learning from a dataset where only a subset of examples are positively labeled).
This was not Dr. Li’s first appearance at ICDM. In November of last year, Li was invited to present his paper, “Deep Heterogeneous Autoencoders for Collaborative Filtering” which proposed a model to address scant data issues of recommender systems and methods to provide more efficient, personalized recommendations, content and services to users.
We are excited to work with Li to integrate many of the novel ideas and innovative models that he learned about in our research and continue to improve our Rakuten ecosystem.
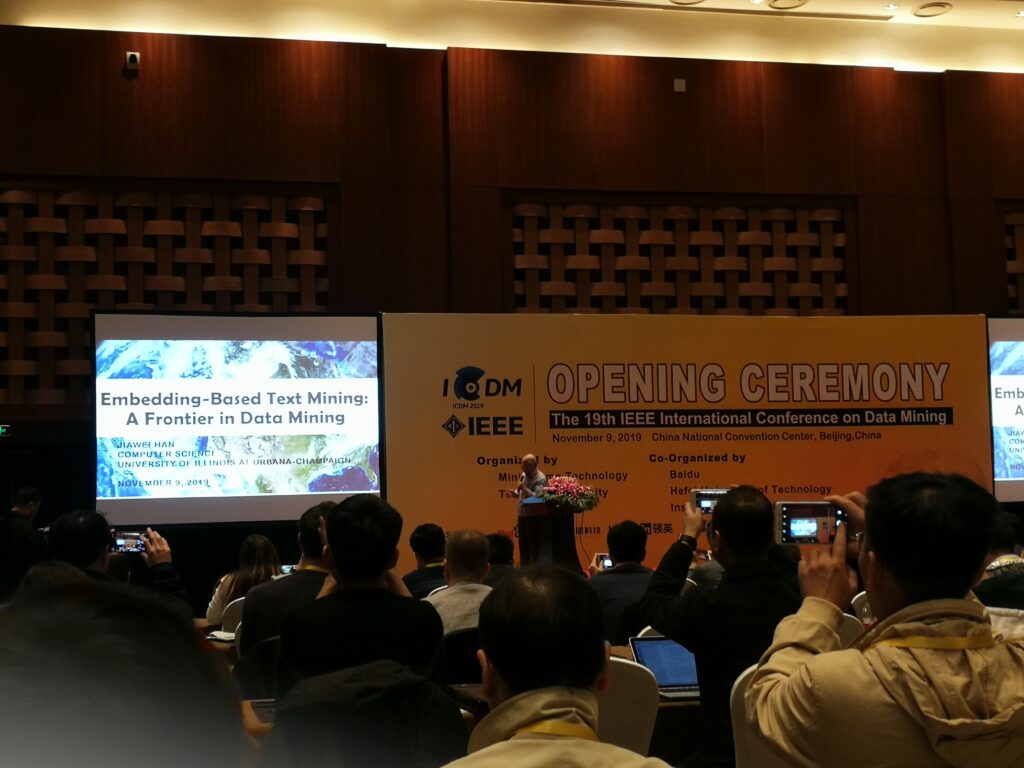