NEWS
Deep Heterogeneous Autoencoders at the IEEE International Conference on Data Mining
In November of 2018, Tianyu Li, a Rakuten Institute of Technology researcher focusing on applied behavior analysis, attended the IEEE International Conference on Data Mining (ICDM) to present his paper. ICDM has become one of the most prestigious conferences in the data mining area and it serves as a significant platform for data miners to share promising research work, attracting attention from both academia and industry. Getting a paper accepted to the conference is very difficult, with high quality standards, and less than 20% of submitted papers this time around got accepted. Because of this we are very happy to get the opportunity to present it to some of the top minds in the field.
The conference covers a wide range of topics, including deep learning, supervised/unsupervised/semi-supervised learning, recommender systems, transfer learning, graph and reinforcement learning. There are always many inspiring and innovative models, methodologies and frameworks presented in each of these topics, so attending is great for widening our views on what is going on in the field, and to bring new ideas back to our daily work.
Although the hot topics are still about complex, powerful deep learning models, more and more research has started to study the interpretability and privacy issue of those models. People have started to think about how to visualize the results of black box algorithms, and how to ensure user privacy when exploiting data. Aside from this, much of the research demonstrated practical applications of transfer learning and reinforcement learning, showing the potential to conduct related research with Rakuten data to achieve more insights and contribution to our services.
For our technologically savvy readers, the Rakuten Institute of Technology paper accepted to the conference is entitled “Deep Heterogeneous Autoencoders for Collaborative Filtering”, and adopts heterogeneous auxiliary information to address the data sparsity problem of recommender systems. It proposes a model to learn a shared feature space from heterogeneous data, such as item descriptions, product tags, and online purchase history. It consists of autoencoders, not only for numerical and categorical data, but also for sequential data, which enables capturing user tastes, item characteristics and the recent dynamics of user preference.
As expected, we got a lot of great feedback after the presentation, giving insights and ideas on how we can keep improving the current work going forward. Another takeaway is that research on real industry-level dataset is getting much more attention than before. One of the reasons is that large scale datasets from industry is essential for current deep learning research. That also means that we, as the research institution of Rakuten, has a lot of potential to conduct cutting edge research, while at the same time contributing to our businesses by applying them using Rakuten data.
For more information on the conference and the paper presented, see the links below.
IEEE International Conference on Data Mining
Deep Heterogeneous Autoencoders for Collaborative Filtering

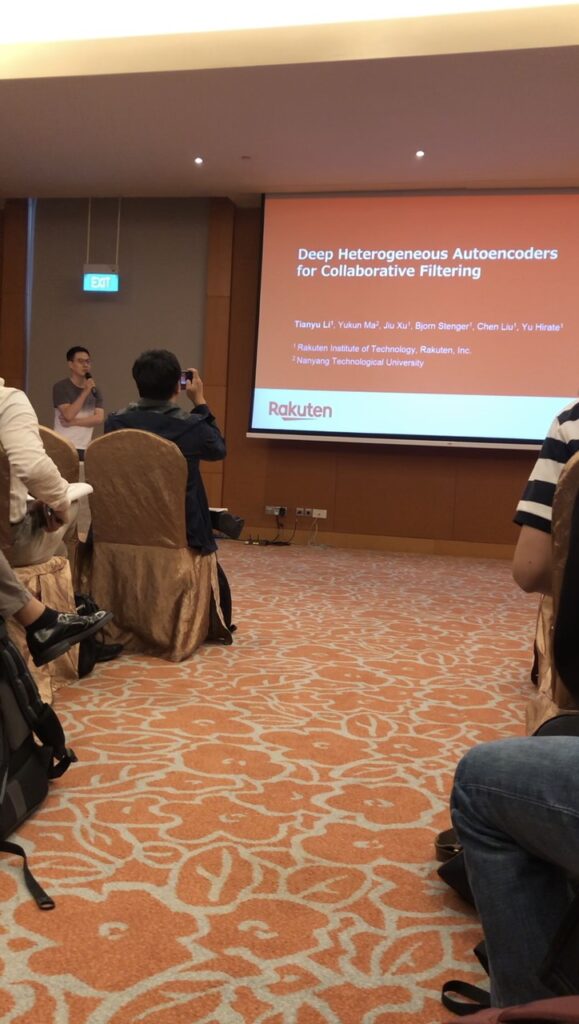